A network of interconnected cubes, often depicted as a diagrammatic representation of a three-dimensional object, can serve various purposes. These networks may visually illustrate the structure and relationships within complex systems, akin to a schematic. Such networks could display connectivity patterns, such as communication links in a network or the arrangement of components in a physical structure.
Visualizations of this type are vital in various fields. For example, in architectural design, they allow for a comprehensive understanding of the spatial arrangement of structures. In engineering, they can model the connections and interactions within systems. Their importance stems from their ability to simplify complex data, providing a clear, concise representation of a system's structure and facilitating intuitive understanding. Historical precedent suggests the use of such diagrams to illustrate mathematical concepts and spatial relationships for centuries.
This understanding of interconnected structures is fundamental to the subsequent exploration of detailed analysis and simulations that comprise the main topics of this article. We will delve into specific applications and limitations of such representations. The following sections will examine how these networks can be used to model, predict, and optimize processes.
Cubes Net
Understanding the structural elements of a "cubes net" is crucial for analyzing complex systems. This network, often diagrammatic, offers valuable insights into connections and interactions.
- Connectivity
- Visualization
- Spatial Relationships
- System Modeling
- Data Representation
- Hierarchical Structures
Connectivity within the network reveals pathways and interdependencies. Visualization simplifies complex data, allowing for easy comprehension of spatial relationships. System modeling employs the network to simulate behavior and predict outcomes. Data representation uses the network as a structured framework. Hierarchical structures, evident in the arrangement of interconnected cubes, enhance understanding of organizational levels. Examples include architectural models showing structural support or circuit diagrams illustrating electronic connections, both showcasing the network's power to visually summarize complex information and enabling the prediction of system performance.
1. Connectivity
Connectivity within a "cubes net" is fundamental. It defines the relationships between individual cubes, representing the flow or exchange of information, resources, or influence. The strength and nature of these connections are critical. A robust, well-connected network exhibits resilience and allows for efficient information propagation, whereas weak or sparse connections can restrict functionality. Consider a network of interconnected architectural cubes representing structural supports: strong connections between cubes ensure stability, while weak connections could lead to structural failure. Similarly, in a network representing communication infrastructure, strong connectivity ensures the reliable transmission of data signals.
The analysis of connectivity within a "cubes net" facilitates the understanding of a system's overall behavior. By examining the patterns of connections, one can identify critical nodes, bottlenecks, or redundant pathways. This is crucial in fields like network engineering, where identifying and mitigating network bottlenecks is vital. Analyzing the connectivity structure can also reveal vulnerabilities in a system, offering proactive strategies for strengthening its resilience and preventing failures. For instance, a network of cubes representing a supply chain's logistics reveals bottlenecks in transportation, leading to improvements in efficiency by strengthening connections between nodes, thereby accelerating product delivery.
Understanding connectivity within a "cubes net" is paramount for optimizing performance and predicting behavior. Identifying and analyzing connection patterns within the network directly impacts decisions for resource allocation, capacity planning, and overall system design. Analyzing the strength and distribution of connections allows for more effective strategies and a more accurate representation of the system's dynamics. The understanding gained from such analysis can significantly inform interventions to improve overall system health, efficacy, and sustainability. This focus on connectivity, therefore, is essential for modeling and comprehending various complex systems.
2. Visualization
Visual representation is intrinsic to the utility of a "cubes net." A well-structured visual model, consisting of interconnected cubes, significantly enhances comprehension of complex systems. This simplification allows for rapid identification of patterns, relationships, and potential issues. The visual nature of the network provides a more accessible understanding compared to purely abstract mathematical models. Architectural designs, for example, use these types of models to represent structural support, allowing engineers and architects to swiftly identify critical load points and potential weaknesses.
The ability to visualize a "cubes net" facilitates more accurate predictions and informed decisions. For instance, in the design of communication networks, visualizing the interconnectedness of nodes allows engineers to understand potential bottlenecks and congestion points, optimizing network performance. Visualizing the relationships within a supply chain network helps managers identify and address inefficiencies in transportation and logistics. Identifying these patterns leads to strategic solutions tailored to specific needs. In essence, "cubes net" visualizations transform complex data into actionable insights. Furthermore, these visualizations offer a clear method to convey intricate information to diverse audiences, including non-technical stakeholders, making the process of understanding and communicating complex systems far more accessible and impactful.
Effective visualization is crucial for managing and interpreting "cubes net" models. Challenges can arise from the complexity of the networks themselves, the sheer volume of data they may contain, and the need to dynamically adjust or update the visualizations to accommodate evolving conditions. However, overcoming these challenges results in powerful tools that enable a deeper understanding of the intricacies and interdependencies within the depicted systems. This capability, in turn, directly contributes to improved decision-making and optimized outcomes in a wide array of fields, ranging from engineering and architecture to supply chain management and logistics.
3. Spatial Relationships
Spatial relationships are integral to understanding "cubes net" models. Accurate representation of relative positions and orientations of cubes is critical for interpreting the model's intended meaning. The positioning of cubes directly conveys information about the structure, connectivity, and interdependencies within a system. This aspect is especially important when interpreting complex systems with multiple interconnected components.
- Precise Positioning and Orientation
Correctly positioned and oriented cubes within the network are essential for accurately representing the spatial arrangement of elements within the system being modeled. This includes precise location and orientation relative to other cubes in the network. Errors in positioning can lead to misinterpretations of the structure, relationships, and functionality. A misaligned cube in a structural model might imply a structural deficiency or instability not reflected in reality.
- Hierarchical Structure & Levels
The spatial arrangement often reflects hierarchical structure, allowing users to quickly identify different levels of organization. Higher-level components might be represented by larger cubes, while lower-level components might be smaller. The placement of these cubes in relation to each other highlights the interdependencies between different organizational levels. This is evident in organizational charts where higher management positions are visually separated from lower-level employees.
- Connectivity & Interaction Visualization
The relative spatial positions of cubes often illustrate the nature and strength of the connections between elements. Proximity might indicate a strong relationship, while separation might imply a weaker interaction. This enables rapid identification of important connections and potential bottlenecks within the system. In transportation networks, closeness of cubes could signify frequently used routes, while distant cubes could represent sparsely used or indirect connections.
- Predictive Modeling and Simulations
Accurate spatial representation within a "cubes net" directly impacts the predictive accuracy of simulations and models based on it. Changes in positions or relationships can be instantly visualized and interpreted, enabling efficient identification of potential system vulnerabilities or inefficiencies. Modifications to a structure, such as a bridge or building, can be immediately represented and simulated through adjustments to the spatial relationships in the corresponding cubes net.
In conclusion, accurately representing spatial relationships within a "cubes net" is paramount. It significantly improves model interpretability and allows for more insightful analysis, more accurate predictions, and a greater understanding of the underlying system's structure and functionality. The effectiveness of the network in conveying information hinges directly on the clarity and precision of these spatial relationships. This crucial factor underlines the necessity of precise and accurate spatial modeling for effective analysis and communication through "cubes net" visualizations.
4. System Modeling
System modeling, a crucial component of "cubes net" analysis, involves the creation of simplified representations of complex systems. These representations often employ a "cubes net" structure to depict relationships, interactions, and dependencies among system components. The "cubes net" facilitates a visual interpretation of these relationships. For example, in an architectural model, cubes might represent structural elements, their interconnections simulating load pathways and stability. In a logistics network, cubes could represent warehouses or delivery points, their connectivity detailing transportation routes and potential bottlenecks. This simplified representation enables analysis of the system's behavior under various conditions, making predictions about its functioning under stress or change. The efficacy of the "cubes net" as a system modeling tool depends entirely on the accuracy and completeness of the data it incorporates.
The practical significance of system modeling, using "cubes net" representations, is profound. It facilitates the identification of critical components, strategic decision points, and potential vulnerabilities within the system. This proactive approach anticipates potential issues, allowing for preemptive measures to enhance system performance or reduce risks. In a manufacturing process, for instance, a "cubes net" model might reveal bottlenecks in the production line, providing targeted improvements in efficiency. Similarly, in an economic model, a "cubes net" can pinpoint areas of vulnerability in supply chains, enabling proactive strategies to mitigate risks. By visualizing complex processes, modeling tools provide a structured method for understanding and intervening in these intricate interactions. These models also allow for experimentation and scenario planning, enabling a more informed approach to problem-solving and resource allocation.
In summary, "cubes net" provides a visual framework for system modeling, facilitating a deeper understanding of complex interdependencies. This simplified representation enables predictive modeling, risk assessment, and informed decision-making. A critical aspect of successful system modeling through the "cubes net" structure is the meticulous collection and interpretation of data underpinning the model. While powerful, models depend on the quality of data input. Accurate representation of spatial relationships, the connectivity between components, and the dynamics of interactions form the foundation for effective system modeling. Consequently, the accuracy and validity of conclusions drawn from a "cubes net" model are directly proportional to the quality of the input data and the methodologies employed in its construction.
5. Data Representation
Data representation within a "cubes net" framework is critical. A "cubes net" is more than a visual aid; it's a structured method for organizing and displaying data related to a system's components and interactions. Appropriate data representation is vital for ensuring the model accurately reflects the system's structure and behavior.
- Data Encoding and Mapping
Accurate data mapping is paramount. Each cube or node within the network must correspond to a specific data point or entity within the modeled system. This involves establishing clear relationships between the physical or conceptual attributes of the system's elements and the corresponding data representations in the "cubes net". For instance, in a network representing a transportation system, each cube could symbolize a specific location, encoded with information about its capacity or accessibility, effectively transforming geographic data into a visual structure.
- Data Attributes and Metrics
The "cubes net" must incorporate the relevant attributes or metrics of the system being modeled. These attributes can include size, capacity, cost, or other essential parameters. For example, in an industrial network, cubes might represent manufacturing units, with associated data including production rates, energy consumption, and defect rates. Accurate representation of these metrics ensures the "cubes net" accurately reflects the operational characteristics of the system.
- Relationship and Connectivity Encoding
The connections between cubes represent relationships within the system. These connections can incorporate various data types, such as flow rates, communication bandwidth, or material transportation routes. If a cube represents a warehouse, its connections to other cubes can represent transport paths and flow of goods, thus quantifying the logistical aspects of the system. Effective encoding translates the complex dynamics between elements into a visual and navigable representation.
- Data Aggregation and Reduction
Complex systems often involve significant data volumes. Data aggregation and reduction techniques within the "cubes net" can help visualize and manage these quantities. Hierarchical representation, for instance, might display high-level summaries in larger cubes with progressively more detailed information displayed in smaller, connected cubes. This approach allows for both an overview and a detailed look into the system's components, reducing data overload.
Effective data representation within a "cubes net" transforms complex information into a comprehensible and manageable visualization. This, in turn, significantly improves analysis, facilitates communication of insights, and enhances the predictive capabilities of the model. The accuracy and clarity of the data representation directly affect the reliability and utility of the overall "cubes net" in understanding and modeling complex systems.
6. Hierarchical Structures
Hierarchical structures are inherent in many complex systems, and the "cubes net" model can effectively represent and analyze these structures. The arrangement of interconnected cubes often reflects a hierarchy, allowing for the visualization of relationships between higher-level components and their subordinate elements. This hierarchical structure is vital in comprehending how different levels of the system interact and influence overall behavior.
- Levels of Organization
Hierarchical structures within a "cubes net" illustrate various organizational levels. Larger cubes might represent overarching systems, while smaller, interconnected cubes represent subordinate components. This clear distinction in size and position allows immediate recognition of the relative importance and dependencies between different elements within the system. For example, in a corporate organizational chart, top management might be represented by large cubes, whereas individual employees could be smaller, connected cubes, visually highlighting the chain of command.
- Dependency Relationships
The connections between cubes in a hierarchical network clearly show dependencies. A cube's connection to a larger, higher-level cube implies dependency. A component or process represented by a cube is reliant on another if it is directly linked to a higher-level cube within the network. This visual representation facilitates identification of critical points and dependencies within complex systems. In a supply chain network, a cube representing a factory's operations would likely connect to a larger cube representing the company, highlighting the factory's reliance on the corporation's overarching structure and policies.
- Information Flow & Control
Hierarchical structures in a "cubes net" can illustrate the flow of information and control between different levels. The connections between cubes can indicate the direction of commands, feedback loops, or data transmission. The representation of power and control flows through a hierarchical network is a crucial factor in managing complex systems. For instance, in a financial institution, top management's cube would connect to the cubes representing various departments. This visualization immediately shows the flow of directives from the leadership to individual departments within the institution.
- Decision-Making Processes
Hierarchical structure within a "cubes net" model can highlight the decision-making pathways. The cubes connected to higher-level decision-makers display the channels through which decisions are disseminated or influence is exerted. In the context of a governmental system, cubes representing government agencies would connect to higher-level cubes representing the executive or legislative branches, illustrating the flow of decision-making processes and powers. Analyzing such connections can reveal decision-making bottlenecks or inefficiencies.
The "cubes net" model's ability to incorporate hierarchical structures provides a crucial tool for understanding complex systems. By visually representing the dependencies and relationships between various levels, the model enables a more complete comprehension of how these systems function and interact. This systematic representation becomes essential when analyzing and strategizing for changes or improvements within complex organizations. The implications of recognizing these hierarchical structures in a "cubes net" extend to numerous sectors, including engineering, logistics, organizational management, and many others.
Frequently Asked Questions (FAQ) about "Cubes Net"
This section addresses common queries regarding "cubes net" visualizations and their applications. Answers are provided in a concise and informative manner.
Question 1: What is a "cubes net," and why is it used?
A "cubes net" is a visual representation of interconnected cubes. Used in various fields, it simplifies complex systems by showcasing relationships and dependencies between components. This visualization aids understanding, facilitates analysis, and supports decision-making processes.
Question 2: What types of systems can be modeled using a "cubes net"?
A wide range of systems can be modeled using a "cubes net," including but not limited to: supply chains, communication networks, organizational structures, transportation networks, and architectural designs. The adaptability of the "cubes net" structure allows for diverse applications.
Question 3: How does "cubes net" differ from other modeling techniques?
"Cubes net" provides a visual and intuitive understanding of systems, emphasizing spatial relationships and connectivity. Other methods may focus on quantitative data analysis or abstract algorithms. The visual approach of a "cubes net" aids comprehension and interpretation by diverse audiences.
Question 4: What are the limitations of using a "cubes net"?
While a "cubes net" is a useful visualization tool, limitations exist. Complex systems with exceptionally numerous interconnected components might become difficult to represent visually. Maintaining model accuracy and comprehensibility in these situations requires careful consideration and simplification strategies.
Question 5: How accurate are predictions based on a "cubes net" model?
The accuracy of predictions depends heavily on the quality of data used to create the "cubes net" model and the assumptions underlying the model's structure. For accurate predictions, the model must precisely capture the nuances of system behavior and incorporate reliable data.
Question 6: What are the benefits of utilizing "cubes net" for system analysis?
Benefits of using a "cubes net" include improved comprehension of complex systems, identification of critical components and relationships, better visualization of connectivity patterns, and a more intuitive approach to decision-making. Visualization aids in spotting potential bottlenecks, vulnerabilities, and optimal configurations within the system.
Understanding these FAQs enhances the application and interpretation of "cubes net" visualizations for various modeling and analysis needs. Careful consideration of the model's limitations and assumptions is essential for accurate results. Next, we will delve into practical examples of its application.
Tips for Utilizing "Cubes Net" Models
Effective utilization of "cubes net" models hinges on adherence to specific strategies. These tips provide a structured approach for maximizing the model's analytical potential and minimizing common pitfalls.
Tip 1: Data Accuracy and Integrity. The foundation of a reliable "cubes net" model rests on precise and complete data. Inaccurate or incomplete data directly translates to an inaccurate representation of the system being modeled. Careful data validation and thorough error checking are essential to avoid misleading conclusions and flawed predictions. Employing multiple data sources and cross-referencing information can enhance reliability. For instance, a "cubes net" representing a supply chain would be rendered inaccurate if warehouse capacity data was missing or if shipping times were imprecise.
Tip 2: Model Simplification and Abstraction. Complex systems may benefit from simplification. A "cubes net" model should focus on key components and relationships, abstracting less critical details. Excessively detailed representations can obscure vital insights and make interpretation difficult. The model should prioritize essential elements while maintaining clarity. For example, a "cubes net" depicting an urban transportation system could highlight major routes and intersections without representing every street and individual bus stop.
Tip 3: Clear Definition of Relationships. Precise definitions of relationships between cubes are crucial. Connections should accurately represent the nature of interactions, whether they are flow of goods, communication channels, or information pathways. Explicitly defining these connections ensures the model's clarity and prevents misinterpretations. For example, connections between cubes in a "cubes net" illustrating a manufacturing process should clearly indicate the flow of materials, not be ambiguously labeled as "connections."
Tip 4: Visual Clarity and Hierarchy. A well-structured "cubes net" model prioritizes visual clarity. Utilize appropriate colors, labeling, and spatial arrangement to enhance comprehension and identification of essential patterns. Hierarchical relationships should be clearly depicted through size, color, or other visual cues to distinguish between higher-level and lower-level components. For instance, a "cubes net" illustrating a company hierarchy should visually differentiate upper management from lower-level staff, making organizational structure immediately apparent.
Tip 5: Iterative Refinement. Models should not be considered static; iterative refinement is vital. The model's accuracy should be regularly evaluated and refined based on new information, feedback, and observed system behavior. Regular updating ensures the model remains a useful tool for analysis and prediction. For instance, a "cubes net" modeling a company's production should be updated with production figures and inventory levels to ensure accurate forecasting.
Tip 6: Thorough Documentation. Complete documentation of the model's components, assumptions, and methodology is essential. This ensures the model's replicability and facilitates future analysis and maintenance. Detailed explanations clarify the meaning behind the connections and their significance for the system being analyzed.
Adhering to these tips ensures that the "cubes net" model remains a powerful tool for understanding complex systems. By emphasizing data integrity, model simplification, relationship clarity, visual appeal, iterative refinement, and comprehensive documentation, users can maximize the insights derived from these models.
Subsequent sections will explore specific case studies illustrating the practical applications of "cubes net" models in various fields. This will exemplify the utility of these models and demonstrate how they can generate actionable insights.
Conclusion
This article has explored the multifaceted applications of "cubes net" models. The analysis demonstrates how these visualizations effectively represent complex systems, facilitating understanding through the simplification of interconnected relationships. Key insights highlight the crucial role of data accuracy, model simplification, and the clear definition of relationships in creating valuable, predictive models. The ability to visualize hierarchical structures within the network significantly improves the understanding of dependencies and information flow. Furthermore, this visual approach offers actionable insights for managing resources, optimizing processes, and predicting outcomes in diverse fields ranging from architecture and engineering to logistics and organizational management.
The potential of "cubes net" models extends beyond immediate applications. Continued research and development in this area promise even more powerful tools for understanding and intervening in complex phenomena. The ongoing need for accurate, insightful representations of intricate systems underscores the enduring importance of such visualizations. The careful application of "cubes net" methodologies, coupled with meticulous data collection and analysis, will undoubtedly contribute to better decision-making and improved outcomes in various domains. Ultimately, the exploration and application of "cubes net" models represent a significant step toward enhanced comprehension and management of the intricate systems that shape our world.
You Might Also Like
Mariska Hargitay: Latest News & UpdatesMario Lipez: Expert Advice & Insights
Michael Joran: Missing Teenager Case Update & News
Does AT&T Own Dish Network? The Answer!
Jack Griffo: Latest News & Updates
Article Recommendations
- Short Positive Quotes About Life Challenges
- Rodney Alcala On Dating Game Video
- Natural Hairstyles Crossword
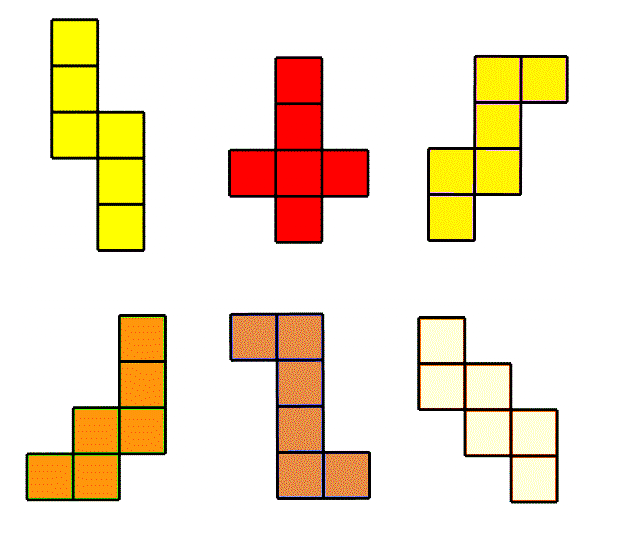
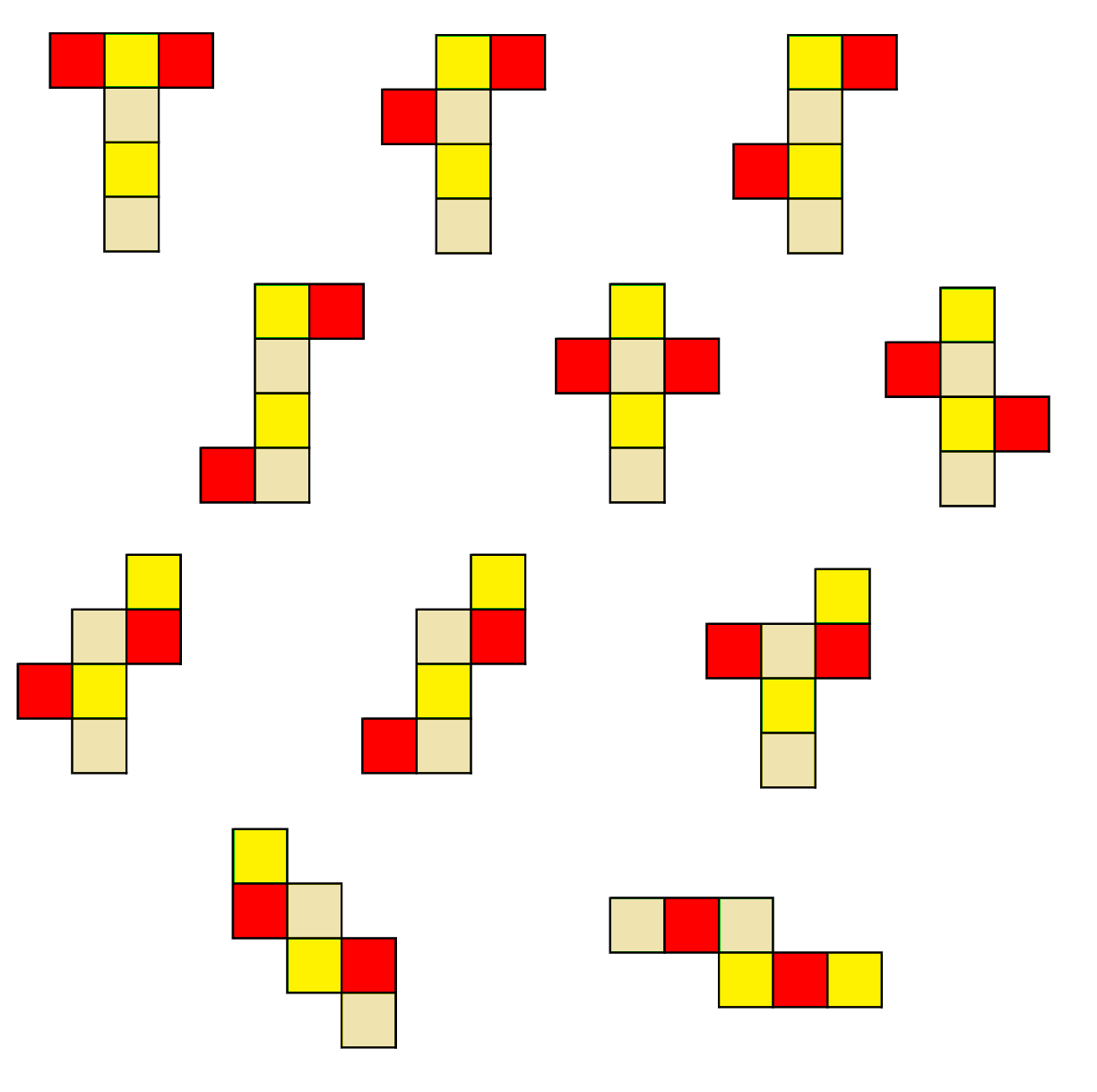
