Individuals whose names begin with the letter "B" encompass a diverse population. Examples include, but are not limited to, individuals named Barbara, Benjamin, Brandon, and Beatrice. This characteristic, while seemingly insignificant on the surface, can be a useful identifier in specific contexts, such as demographic studies, name-based sorting, or in certain databases where a consistent alphabetical ordering is required. It is important to remember that the inherent significance of this identifier is contingent on the specific research or application in question, and is not a defining trait in general population studies.
The practical applications of grouping individuals by initial letter, including the letter "B", are often limited. The value is frequently contextual. For instance, in alphabetized lists or data analysis requiring simple sorting, such a classification system might be beneficial for streamlining tasks. However, it rarely offers deeper insights into the characteristics or behaviors of those included in the group. The approach should not be taken to suggest a correlation between shared initial letter and particular attributes. Further research or deeper analysis is necessary to establish any meaningful connections.
This discussion of individuals whose names begin with the letter "B" sets the stage for a more in-depth exploration into the broader topic of alphabetical organization in data handling. We will then move on to examine the practical implications and potential pitfalls of such arrangements, considering their relevance to modern data management strategies and their limitations in drawing significant conclusions about populations.
People Starting with B
Categorizing people based on the initial letter of their names is a rudimentary yet sometimes necessary approach in data management and analysis. This exercise, though simple, can reveal patterns, assist in sorting, and serve as a starting point for deeper investigations.
- Name recognition
- Alphabetical order
- Data organization
- Demographic sampling
- Statistical analysis
- Limited insights
Name recognition is crucial for initial identification. Alphabetical order aids in data organization. Demographic sampling can utilize initial letters, but not effectively. Statistical analysis might initially use this as a preliminary grouping method, but its usefulness is limited. While this categorization can be a quick way to sort data, it's not a robust method for deriving meaningful conclusions about people. For example, grouping individuals by "B" reveals little about their collective traits, professions, or experiences. The approach's success hinges on the specific context and goals of the investigation.
1. Name recognition
Name recognition, in the context of identifying individuals whose names begin with the letter "B," serves as a fundamental initial step. This process, while basic, is crucial for the subsequent organization and potential analysis of data related to such individuals. Its value stems from its role in streamlining identification and retrieval within structured datasets.
- Basic Identification
Name recognition facilitates the straightforward identification of individuals whose names begin with "B." This process is essential in sorting lists, databases, or other organized collections of people. For instance, in a roster of students, quickly identifying students with names starting with "B" allows for efficient targeting and retrieval of specific data.
- Data Filtering
Name recognition can be a vital filtering tool for extracting particular subsets from a larger dataset. If an investigation necessitates examining information pertaining solely to individuals with names starting with "B," this initial recognition step streamlines the process. Examples include retrieving all student records, employee records, or patient records from a database that match this criteria.
- Initial Sorting & Categorization
In pre-processing stages of data analysis, recognizing names starting with "B" enables straightforward initial sorting and categorization. This is particularly important in initial stages of broader data analyses when organizing data efficiently is paramount. Such sorting often facilitates the subsequent application of more sophisticated analytical techniques.
- Limitations in Deeper Analysis
It is crucial to acknowledge that name recognition based solely on the initial letter, while useful for basic identification, has limitations. This method does not provide insights into individual attributes, characteristics, or relevant demographics. Correlation or conclusions based solely on the initial letter of a name are not valid and should not be used in deeper analysis, and require further investigation or data sources for valid results.
Name recognition, therefore, plays a crucial initial role in handling data related to individuals whose names start with "B." It efficiently identifies and isolates individuals within larger datasets, enabling more targeted and manageable investigations. However, it is imperative to recognize that it remains a superficial characteristic and lacks the capacity for comprehensive insights, underscoring the need for more in-depth analysis. Any conclusions drawn from this limited facet must be approached with caution and should be further supported with additional evidence from more robust analysis methods.
2. Alphabetical Order
Alphabetical order, a fundamental organizational principle, plays a role in managing data related to individuals whose names begin with the letter "B." Its application dictates the sequence in which information is presented and retrieved. This structured arrangement, while seemingly simple, has practical implications for data management and analysis within various contexts. The significance of alphabetical order in this specific case lies in its ability to organize and locate information efficiently.
- Data Management and Retrieval
Alphabetical order facilitates swift data retrieval. In a list of names, locating individuals beginning with "B" becomes straightforward. This systematic organization is essential in databases, lists, and other structured data collections. The process is particularly relevant when information about people starting with "B" needs to be accessed or updated efficiently.
- Data Sorting and Analysis
Data sorting based on alphabetical order allows for systematic analysis. While potentially limited for comprehensive population-level insights, alphabetical sorting can prove beneficial in preliminary data exploration. This step might precede further, more sophisticated analyses. This ordered presentation of data aids in identifying patterns or trends in a subset of individuals.
- Database Organization
Within databases containing personal information, alphabetical order for names is a common practice. This ensures consistent and predictable retrieval of data related to individuals whose names begin with the letter "B." Maintaining order in such databases is crucial for ensuring accurate and efficient access, modification, or deletion of information. Specific database search functionalities frequently rely on alphabetical search.
- Limited Predictive Value
Crucially, the alphabetical ordering of names associated with individuals starting with "B" offers limited predictive capability or insights into their attributes, characteristics, or shared experiences. Such ordering is simply a sorting tool, and should not be interpreted as indicating any inherent connections or correlations between the letter "B" and other features.
In summary, alphabetical order serves as a fundamental organizational tool in the context of identifying and managing information about individuals beginning with "B." Its primary value lies in facilitating efficient data retrieval and preliminary data analysis. However, it's essential to understand that relying solely on alphabetical order is insufficient for drawing meaningful conclusions or inferences about the individuals in question. Deeper, more complex analysis techniques are necessary for such objectives. More comprehensive methods for gathering and interpreting information about populations, are essential for drawing valid and useful conclusions.
3. Data Organization
Data organization, when applied to individuals whose names begin with "B," involves arranging and structuring information pertaining to those individuals. This process might encompass names, contact details, demographic data, or other relevant information. The efficiency of data retrieval and analysis hinges significantly on the effectiveness of this organizational structure. Effective data organization allows for quick identification and retrieval of specific information related to individuals within this subset. Without proper organization, the process becomes cumbersome and prone to errors.
Real-world examples of this principle include student databases, employee rosters, or patient records. In these contexts, organizing data alphabetically by the initial letter of an individual's last name, or in this case, the first letter, enables fast searches. For instance, in a student database, locating all students with names beginning with "B" requires a structured approach, such as alphabetical sorting. Similarly, in a large organization, finding employees with last names beginning with "B" becomes significantly more efficient within a structured and sorted database.
The practical significance of understanding this connection is multifaceted. Efficient data organization ensures timely access to information, reducing delays in processing requests or tasks. This efficiency translates into improved productivity and cost-effectiveness. Further, a structured system for organizing data pertaining to individuals whose names begin with "B" supports the development of informed decisions, particularly in contexts requiring specific analysis of this group. Accurate and systematic data organization underpins these benefits. While seemingly rudimentary, this organizational approach is pivotal in various applications, underscoring the fundamental importance of structured data management in contemporary environments.
4. Demographic Sampling
Demographic sampling, a technique used in research, involves selecting a subset of a population to represent the larger group. The connection between this methodology and individuals whose names begin with "B" is limited and largely inconsequential. Demographic sampling aims to gather data representative of broader characteristics of a population, not to focus on a specific initial letter of a name.
While selecting a sample based on names beginning with "B" could occur in a small-scale study, its usefulness is practically negligible. For instance, a small research project examining the spending habits of individuals named Beatrice may employ a sample of people whose names begin with "B." However, the findings likely lack generalizability to the broader population. Such a sample would be severely limited in its ability to reflect the experiences or spending patterns of other individuals within a larger population due to the small subset represented by the initial letter. Drawing inferences about larger trends from a group identified solely by their initial letter would be an invalid approach.
The lack of meaningful connection between demographic sampling and individuals whose names start with "B" underscores the importance of carefully defining the population of interest and the variables being examined. Researchers must employ appropriate sampling techniques to gather data relevant to the broader population, ensuring the findings are reliable and applicable to the target group. Inaccurate or inappropriate sampling methods can lead to misleading conclusions. Furthermore, a focus on the initial letter of a name introduces a highly insignificant and statistically meaningless variable, diminishing the validity of any subsequent analysis. Valid demographic research depends on careful selection methods, not name prefixes.
5. Statistical Analysis
Statistical analysis, while a powerful tool for understanding populations, offers limited value when applied solely to individuals categorized by the initial letter of their names, such as "B." Categorization by initial letter does not inherently possess statistical significance. This approach typically lacks the necessary breadth and depth of data to support meaningful conclusions. Any observed patterns or correlations within such a limited subset are likely spurious and not indicative of broader trends.
- Limited Data for Generalizations
Statistical analysis requires a substantial dataset to draw reliable inferences. Classifying individuals solely by the initial letter of their names produces a dataset far too limited for meaningful statistical analysis. A small sample size, in this case, compromises the validity of conclusions and prevents the identification of trends generalizable to a larger population. For example, analyzing the income levels of individuals named "Benjamin" will not reliably represent the income distribution within the broader population. A small sample group is not a suitable basis for conclusions about the entire population.
- Potential for Spurious Correlations
Statistical analysis aims to identify correlations between variables. Grouping people by an arbitrary characteristic like the initial letter of their names can lead to spurious correlations. Observed patterns within this restricted group may not reflect underlying relationships in the broader population. For instance, if individuals named "Beatrice" disproportionately pursue careers in a particular field, the relationship may be a coincidence unrelated to the initial letter and not a genuine correlation.
- Misinterpretation of Results
Analyzing data based on such a superficial grouping can lead to incorrect conclusions. Any detected patterns are not necessarily indicative of meaningful relationships. Results derived from this approach need extreme caution and further investigation with a broader dataset. Misinterpretations might suggest false associations and correlations between the initial letter of a name and other variables, misdirecting subsequent analysis.
- Alternative Approaches for Insights
More insightful statistical analysis requires data on relevant variables. To gain a clearer understanding of a population, researchers should collect data on characteristics directly related to their research question, not the initial letter of a name. For instance, investigating the relationship between occupation and education level demands comprehensive data on both fields, not a simple categorization based on the initial letter of a name.
In summary, statistical analysis, when applied to groupings based solely on the initial letter of names, such as "B," poses significant challenges to drawing valid inferences. The limitations inherent in the small and arbitrary sample size render the results unreliable. Meaningful statistical analysis necessitates robust data encompassing relevant variables, not superficial name-based classifications.
6. Limited Insights
Categorizing individuals solely by the initial letter of their names, such as "B," yields limited insights. This approach provides a superficial grouping, lacking the depth required for meaningful analysis. The inherent limitations of this methodology become evident when considering the diverse range of characteristics, experiences, and behaviors that define individuals.
- Superficial Grouping
Categorization based on the initial letter of a name is a rudimentary method. It fails to capture the complex interplay of factors shaping individual identities. An individual's name is a superficial identifier, offering no meaningful insight into their socioeconomic status, professional achievements, or personal attributes. This surface-level classification obscures crucial details.
- Inadequate Data Representation
Using initial letters for grouping produces an extremely limited dataset. This limited sample size hinders the ability to draw statistically significant conclusions or generalize findings to a broader population. Such a narrow focus on a single, arbitrary characteristic fails to account for the myriad factors that influence an individual's characteristics and behavior.
- Absence of Contextual Understanding
The initial letter of a name lacks inherent contextual meaning. This approach fails to account for the multifaceted nature of individuals. It ignores crucial demographic information, lifestyle choices, and other elements essential to forming a comprehensive understanding. Any inferences drawn based solely on this limited identifier are potentially inaccurate.
- Potential for Misinterpretation
Grouping individuals based on such a superficial criterion can lead to misinterpretations or erroneous conclusions. Any observed patterns within the limited subset might not represent broader trends or relationships. Attributing specific traits to a group based solely on their names can lead to inaccurate judgments.
In conclusion, focusing solely on individuals whose names begin with "B" provides severely restricted insights. The approach is inadequate for drawing meaningful conclusions about a population. Understanding the inherent limitations of this method is crucial for avoiding misinterpretations and ensuring sound data analysis. More comprehensive methodologies must be employed to obtain valid and accurate results when examining complex characteristics of populations.
Frequently Asked Questions about Individuals Beginning with "B"
This section addresses common inquiries regarding individuals whose names start with the letter "B." The questions and responses aim to clarify potential misconceptions and provide accurate information.
Question 1: Does grouping people based solely on their first letter, like "B," have any statistical significance?
Answer 1: No. Such grouping lacks statistical significance. A small and arbitrary sample like this, focused on a single, non-essential characteristic, does not allow for valid generalizations about broader population trends. Statistical analysis demands robust datasets encompassing a wider range of variables to derive reliable correlations.
Question 2: Can demographic data be reliably collected or analyzed by only considering individuals starting with "B"?
Answer 2: No. Using a limited dataset constructed solely on name prefixes for demographic study is insufficient. Demographic analysis requires comprehensive data representing a diverse range of characteristics to ensure accurate representation of a population.
Question 3: Are there any instances where classifying people by their initial letter, such as "B," is useful?
Answer 3: In certain, very specific contexts, initial letter categorization might be a practical first step for preliminary sorting in data management. However, this approach remains superficial and inadequate for in-depth analysis or drawing broader conclusions about a population. Examples include initial list filtering or basic data sorting procedures.
Question 4: Does having a name starting with "B" indicate any particular personality traits or characteristics?
Answer 4: No. Associating personality traits or characteristics with a single letter of a name lacks factual basis. Individual characteristics are complex and shaped by numerous factors, not a single, arbitrary identifier.
Question 5: Can this method of initial letter grouping inform insights into collective experiences or behaviors?
Answer 5: No. Such an approach yields insufficient insight. The method is too simplistic to reliably reflect shared experiences or behaviors within a group.
Question 6: How can individuals avoid misinterpreting results based on superficial categorizations like initial letter groupings?
Answer 6: Researchers should carefully consider the limitations of such classifications. Approaches focusing only on superficial identifiers are not sufficient for sound conclusions. A deeper, more thorough analysis that encompasses a broad range of relevant variables is crucial to ensure accurate interpretations of data.
In summary, while initial letter categorization, like "B," might have limited applications for rudimentary data organization, it cannot be used as a foundation for meaningful analysis of complex populations or individual traits. Statistical significance, accurate representation, and robust data are essential for reliable conclusions.
Moving forward, this discussion underscores the importance of carefully chosen and comprehensive methodology in data analysis.
Tips for Effective Data Handling and Analysis
This section outlines practical advice for managing and analyzing data, particularly when focusing on individuals whose names begin with a specific letter. The following tips emphasize the importance of rigorous methodology and accurate interpretation to avoid drawing erroneous conclusions from limited data.
Tip 1: Data Collection and Quality. Thorough data collection is paramount. Data must be complete, accurate, and relevant to the research question. Inaccurate or incomplete data, regardless of the initial letter of a name, severely compromises the reliability of analysis. Verification and validation processes are essential to ensure the quality and integrity of the data set.
Tip 2: Sample Size and Representativeness. A large and representative sample is crucial for generalization. A small sample drawn from a limited group based solely on the initial letter of names is insufficient to reflect the broader population. Using appropriate sampling techniques is essential to obtain results applicable to the entire population, rather than a limited subset.
Tip 3: Careful Selection of Variables. Only include variables directly relevant to the research question. Focusing on superficial identifiers like the initial letter of a name is insufficient. Include relevant demographic information, attributes, and behaviors. Relevance to the research question is critical for sound conclusions.
Tip 4: Statistical Significance and Robustness. Employ appropriate statistical methods and assess the statistical significance of any observed patterns or trends. Avoid drawing conclusions from spurious correlations based on limited datasets. Statistical tests should be chosen carefully to align with the study design.
Tip 5: Contextual Understanding. Place findings within their proper context. Recognize the limitations of using a single variable, such as the initial letter of a name, to draw conclusions. Contextual awareness is crucial to avoiding misinterpretations and forming accurate judgments about broader patterns or trends.
Tip 6: Avoiding Confirmation Bias. Researchers should be mindful of potential biases influencing the interpretation of data. Subconscious biases can affect the selection of variables or the interpretation of results. Employ objective and impartial methods to ensure accurate conclusions. Critically evaluating assumptions is vital to mitigate bias in the research process.
Tip 7: Transparency and Documentation. Clear documentation and transparency regarding data collection, analysis methods, and conclusions are essential. This enables others to critically assess the study's methodology and results. Documenting decisions made during analysis helps to maintain objectivity and accountability.
Following these practical tips ensures the integrity of data analysis, regardless of the specific group being studied. Rigorous methodology and a keen awareness of limitations are essential to avoid drawing erroneous conclusions from limited datasets.
This section lays the groundwork for subsequent discussions, highlighting the importance of sound methodology in data analysis. The following sections will delve into the specifics of advanced data analysis techniques applicable to various research areas. Crucially, the need to avoid superficial analysis is highlighted and emphasized throughout.
Conclusion
The exploration of individuals whose names begin with "B" reveals the limitations of superficial categorization. While initial sorting by the first letter of a name might be practical for basic data management, it lacks the statistical robustness and contextual depth to yield meaningful insights into the characteristics or behaviors of the group. The analysis emphasizes the need for comprehensive data collection and analysis techniques, including consideration of a broad range of variables beyond this simple identifier. This method proves inadequate for generalization to broader populations and can potentially lead to misinterpretations.
The article underscores a critical point: superficial analysis can lead to misleading conclusions. Meaningful understanding of populations requires moving beyond simplistic groupings and embracing sophisticated methodologies. Future research should prioritize robust data collection, appropriate sampling techniques, and rigorous statistical analysis to avoid spurious correlations and achieve accurate results. The focus should remain on the relevant variables and characteristics that define populations, rather than arbitrary initial letters of names.
You Might Also Like
Patient Customers, Thank You!Hilarious Alligator Memes!
Dallas Storage Auctions: Top Deals & Finds!
Paul Cerrito Net Worth 2024: Updated
Happy Birthday To This Amazing Woman! A Special Day
Article Recommendations

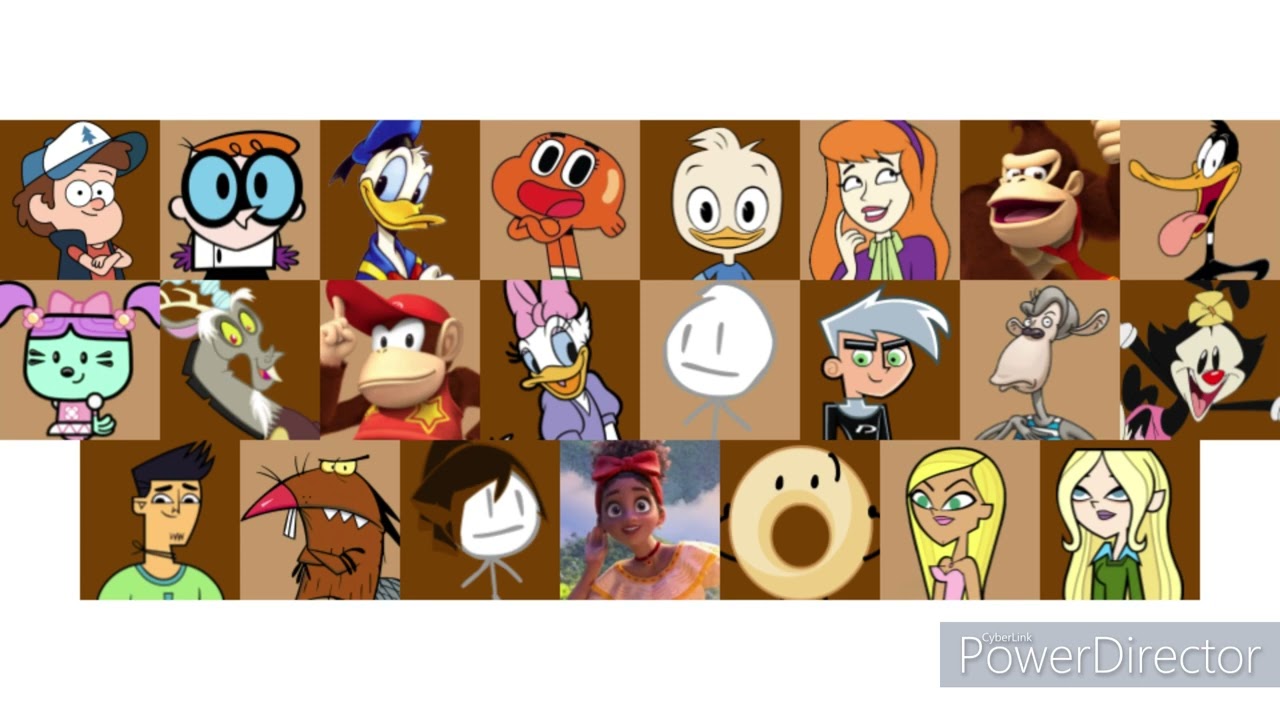
